Background
Before I can share a bit about the promising results of our latest article published in the Journal of Sports Science, I consider it important to highlight the reasons and motivations that led me to pursue my PhD on the training monitoring and individualization topic in the first place. As a former under-18 soccer player, I was always intrigued about training adaptation and how it occurs, but more importantly why the training prescription was continuously developed in a very rigid way, without considering how I was both physiologically and especially psychologically fit to perform. Of course, it was a very naive thought at that time and with no scientific background but was a starting point that led me to the fascinating academic world. A few years later, with more scientific knowledge, and still with the curiosity to improve training adaptation through a more efficient training intensity prescription I began my PhD project, from which I am avid to share the initial results.
Training monitoring and prescription: Is there any difference between using objective and subjective measures as decision-making tools?
Athletic preparation has remarkably developed in terms of scientific approach. The spurious growth of science-led training in the last decade should be attributed to the vast research done on athlete monitoring and training prescription individualization. Scientific training continues to be one of the hottest topics in sport science. It is already well established that a well-designed monitoring system can manage training load and recovery to optimize performance capacity by providing the right stimuli at the right time and avoiding deleterious outcomes like overtraining (8,13).
Likewise, it is already known that high-intensity interval training (HIIT) is a good training stimulus to improve endurance training adaptations by accumulating higher amounts of training at or close to VO2max (i.e., > 90{32c02201c4e0b91ecf15bfd3deecd875caca8b9615db42cfd45ce3d8de8d0829} of VO2max) (3). As already professed by HIIT Science, at least twelve variables could be manipulated during different HIIT sessions, from the number of intervals to between series recovery (Fig. 1). However, we could also include the timing of its prescription as a manipulation variable, which has become a key variable to consider when programming HIIT. All the HIIT manipulation variables regarding the series (Box 1), sets (Box 2) and physiological response-altering interventions (Box 3) could be adjusted properly, but performance would be compromised if the athletes are not ready to take that amount of effort on that particular day. Therefore, an athlete’s readiness should also be considered as another group of variables influencing the physiological response and adaptations to HIIT (Box 4).
The most common athlete monitoring systems can include either or both “objective” and “subjective” measures. The former is more frequently used and typically involves wearable technology to measure various surrogates, such as athletic performance, which includes maximal oxygen uptake, muscle force and power measures, heart rate-related variables, and submaximal fitness tests (19). The latter provides insights regarding the psychological and psychobiological factors related to internal factors such as mental fatigue, muscle soreness, perceived stress symptoms and well-being typically measured through questionnaires (6). Accordingly, an increase in the use of objective measures such as heart rate variability (HRV), as a tool to individualize HIIT training has gained popularity, as it demonstrated to be more effective when compared to more rigid training programming (10,11).
So is there any difference between objective and subjective measures?
Interestingly a previous systematic review conducted by Saw (18) has demonstrated that subjective measures are more sensible, consistent, and more responsive and trump commonly objective measures like HRV to report acute and chronic training loads.
What is the possible mechanism behind that?
It has been recognized that the stressors associated with performance are quite varied and originate from outside as well as within the sports environment (16). Whilst originated from different aspects, the intricated cascade of events that occur once the brain detects a disruption in homeostasis caused by stressors is very similar, which is characterized by augmented secretion of norepinephrine and epinephrine by the sympathetic nervous system (SNS) as well as the release of the hypothalamic-pituitary-adrenal (HPA) axis hormones (corticotropin-releasing hormone, adrenocorticotropic hormone and cortisol).
Stressors from training play a major role in training adaptation, but as soon as the athletes leave the track, pool, or pull over their bike at home, the training stressor ends, and they switch to the recovery process. However, more often than not, various life stressors come into place, influencing the ability of an athlete to recover and perform well at the next session. Thus, the principal difference between stressor responses may be the frequency of exposure. Whether it’s daily life or programmed training stress, the nervous system discharges the same stress response cascade. With this thought in mind, chronic exposure to both types of stressors could lead to one or more forms of SNS saturation and HPA axis dysregulation, which may complicate the training response interpretation when solely using objective measures, leading to training intensity prescription errors.
This is evidenced by studies showing increased power output at the same submaximal heart rate (HR) in cyclists associated with both decreased and increased training status (4,7). These responses may indicate one of two things: a) cyclists are adapting to training, as they produce more power at the same submaximal HR, or b) an increase in the parasympathetic nervous system (PNS) activity as a protective effect, limiting the engagement of the sympathetic system for increasing HR during exercise, reflected by the need to exercise at a much higher workload to reach the target heart rate (Fig. 2). Therefore, considering only common objective measures evaluated in submaximal tests, without a holistic appreciation of the athlete’s neuropsychological status, it is not possible to discern between these two training responses.
More interestingly, subjective measures like rating of perception of effort (RPE) during the submaximal test, and the DALDA questionnaire were able to differentiate both training statuses among cyclists (4,7), giving more context to training responses, again confirming the higher sensitivity of these measures. Cyclists when exercising at higher workloads for the same HR while fatigued will likely report a higher perception of effort or will be less tolerant to training and non-training stressors.
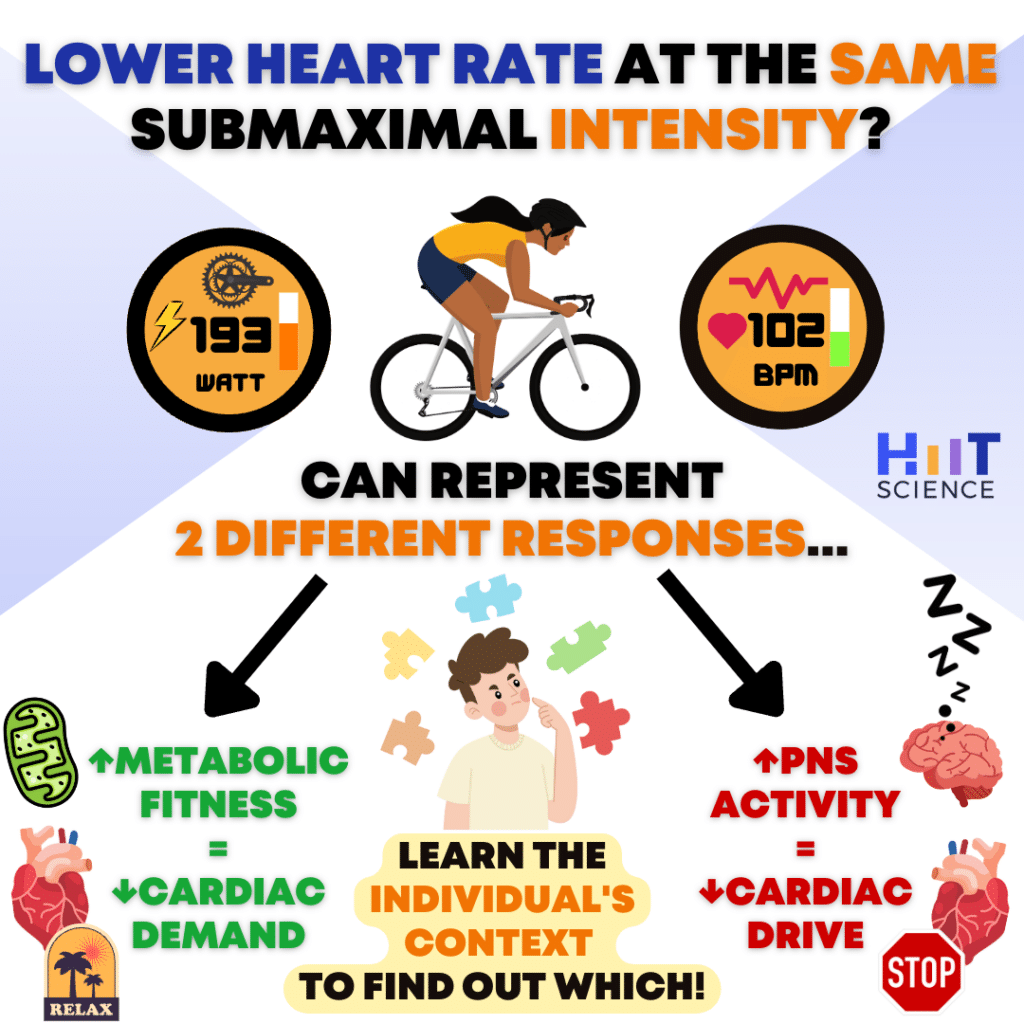
Surprisingly, self-reported measures of stress tolerance have been used only for training monitoring purposes rather than as an indicator that could help guide decision-making. Moreover, few studies have attempted to individualize HIIT prescriptions considering subjective measures (14).
Our recent work (8) sought to investigate the role of self-reported measures of stress tolerance not only as a tool to monitor how recreational runners were responding to training but also as a tool for individually prescribing training intensities on a daily basis and their effect on improving endurance performance.
The research
The study aimed to examine the effect of endurance training individually guided by an objective (HRV) or self-reported measure of stress (DALDA questionnaire) compared to a predefined endurance training prescription for increasing running performance.
After 2-weeks of measurements to determine both HRV and DALDA baseline values thirty-six male recreational runners were randomly split into three groups, HRV-guided training group, DALDA-guided training, and a predefined endurance training prescription group. Endurance performance was evaluated before and after a 5-week training intervention.
How training was programmed?
The training sessions for all groups consisted of moderate-intensity continuous training (MICT) and high-intensity interval training (HIIT). Training sessions and periodization of the predefined group were structured in a way that there were no changes throughout the experimental protocol. Meanwhile, the periodization for HRV guided group, especially the HIIT was prescribed based on the 7-day moving average of lnRMSSD (lnRMSSD7day), which was compared to the threshold determined during the baseline period (the smallest worthwhile change- SWC) (15), and which has been used in the HRV-guided literature (10,11). Thus, values of lnRMSSD7day above or below the SWC were regarded as a negative outcome to training and the intensity changed from HIIT to MICT. For the DALDA training group, the periodization of HIIT was based on the daily DALDA values based on the recommendations of Rushall (16). During the baseline period a “window of appropriate training response” was calculated for “worse than normal” scores, in a way that during training, data points higher than the values included in this “window” indicated an inability to cope with stressors (i.e., lower stress tolerance), resulting in the change of the training intensity from HIIT to MICT. Furthermore, different from previous studies (10,11) all the participants were blinded for their condition.
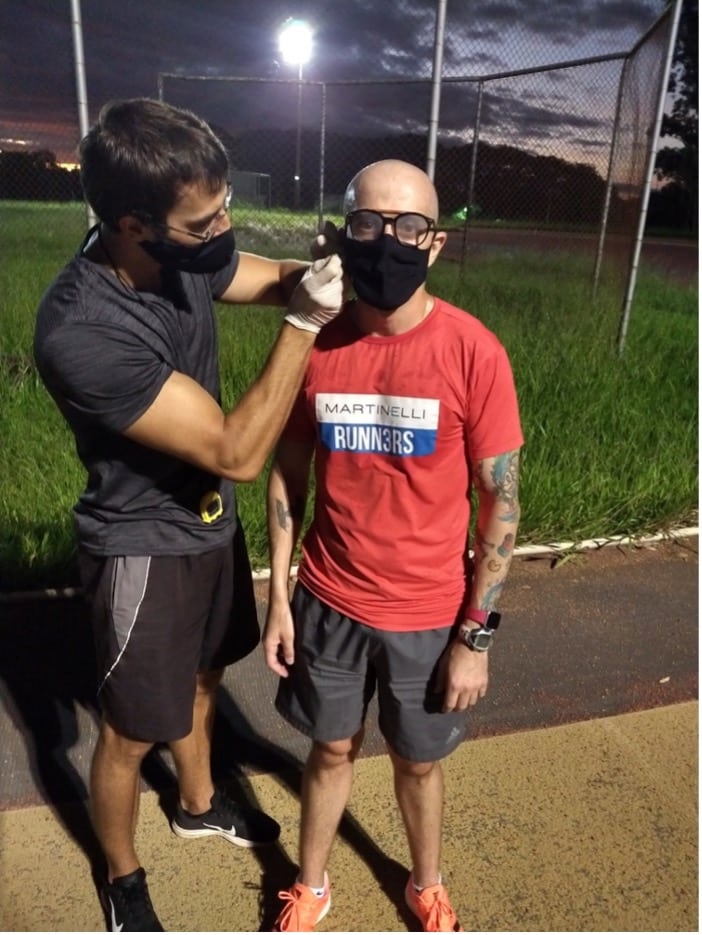
Collecting a blood sample from the ear of one of the participants to evaluate a lactate concentration after a field test at the State University of Maringá running track.
The results
Confirming our initial hypothesis, training individually guided by HRV improved peak velocity (6.6{32c02201c4e0b91ecf15bfd3deecd875caca8b9615db42cfd45ce3d8de8d0829}) and 5km time-trial (-8.3{32c02201c4e0b91ecf15bfd3deecd875caca8b9615db42cfd45ce3d8de8d0829}) to a greater extent when compared to the predefined prescription group (4.9 and -6.0{32c02201c4e0b91ecf15bfd3deecd875caca8b9615db42cfd45ce3d8de8d0829}). Likewise, the DALDA-guided training group was also demonstrated to be more effective for increasing peak velocity (8.4{32c02201c4e0b91ecf15bfd3deecd875caca8b9615db42cfd45ce3d8de8d0829}) and improving 5km time-trial (-12.8{32c02201c4e0b91ecf15bfd3deecd875caca8b9615db42cfd45ce3d8de8d0829}) when compared to the predefined prescription group (Fig. 3).
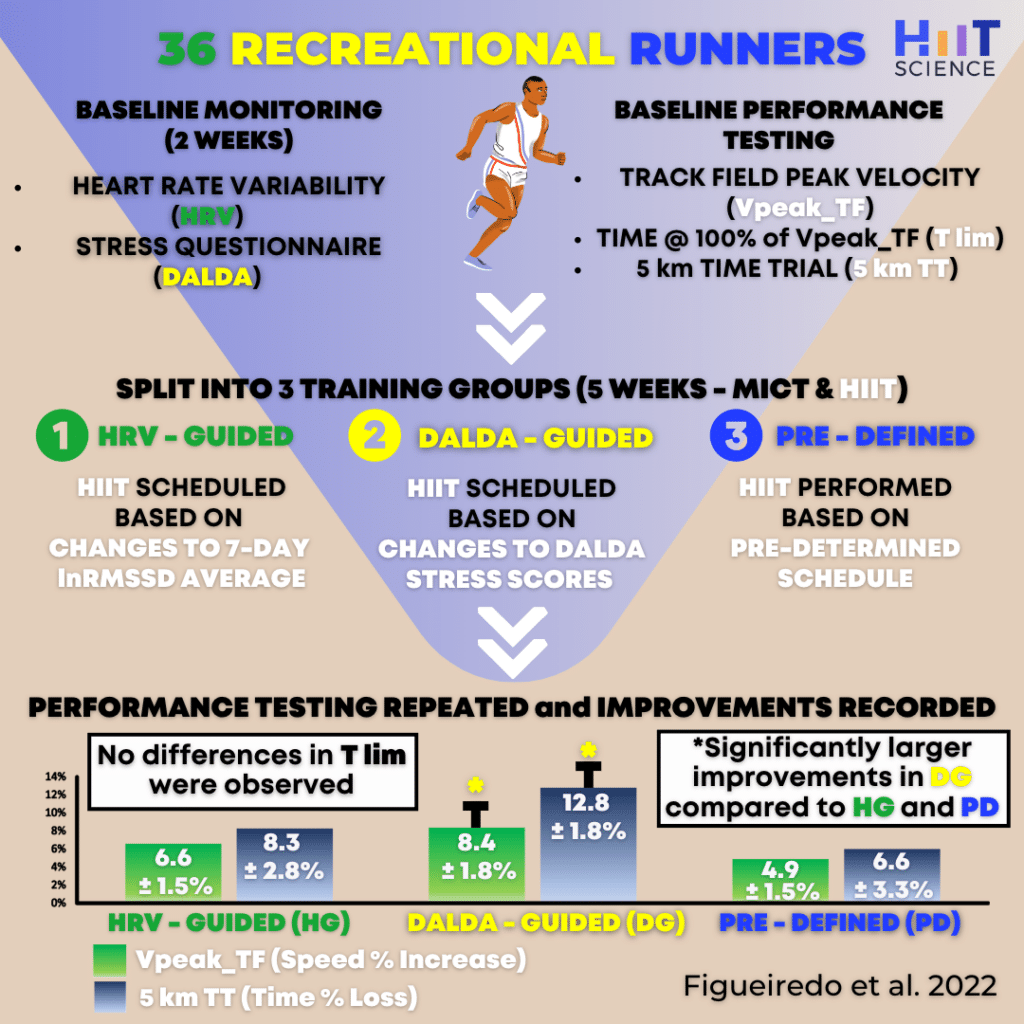
However, interestingly, the DALDA group demonstrated a higher magnitude of change in endurance performance when compared to the HRV group. Moreover, no differences were found in the number of HIIT sessions between groups during the experimental protocol, as well as for the measures of training load, which is very similar to previous findings (10,11,14). Likewise, there were no differences in the proportion of training sessions changed from HIIT to MICT for HRV and DALDA groups (? 12{32c02201c4e0b91ecf15bfd3deecd875caca8b9615db42cfd45ce3d8de8d0829}). Altogether, these results suggest that changes in endurance performance are more related to better timing of training prescription, being HIIT performed under less stress response leading to a reduction in intraindividual variation in training adaptation.
So what could explain the higher improvement found for the DALDA group when compared to HRV guided group?
In a nutshell, considering HRV response and prescribing HIIT only when values were within the SWC might have limited potential increases in performance, due to changes in training prescription (HIIT to MICT, when HRV were above the SWC), when in fact athletes perceived subjectively that they were more tolerant to stressors.
Therefore, prescribing HIIT guided by individual perceptions of training and non-training stressors as evaluated by the DALDA questionnaire could add some context to training by decreasing the possibilities of either false negative – no changes in training content when it should (i.e., HRV within SWC with lower stress tolerance) and false positive changes in training content when it was not deemed necessary (i.e., HRV above SWC with higher training tolerance) errors (2), leading to better training adaptations.
How to incorporate subjective self-reported measures of stress into your training program for an overall decision-making framework?
Even though our results are promising regarding the use of a subjective self-reported measure as a tool to better individualize endurance training intensities on a daily basis, careful consideration must be taken before the effective implementation of this measure in the applied sport setting, with the purpose to minimize possible sources of error. This pertains to how these measures are designed, implemented, and the athlete’s cognitive, and situational factors that might have some influence to obtain reliable and accurate data (17). Not considering these points may result in outcomes that are biased and not truly reflective of self-response of how athletes are or are not tolerating stressors, perceiving effort, and so forth, which may result in misguided management of the training program. All of these points are related to conscious bias (1,17), often associated with a more socially desirable manner to respond to psychological questionnaires, either by over-reporting favourable responses or under-reporting unfavourable responses (13,17), in a way to get the desirable training stimuli.
Therefore, there are some practical actions that can be implemented to reduce the barriers to using self-reported measures in the field and get some good data to be used in a meaningful way, such as:
- The use of validated multiple-item questionnaires with psychometric properties to the detriment of non-validated customized, single-item questionnaires. These single items tools are more susceptible to random measurement errors and to cognitive factors, which include miscomprehension and unknown biases in meaning and interpretation (12,17).
- Avoid collecting self-reported measures of stress with multiple athletes at the same time, or in the same locality to minimize the change of answer comparisons, avoiding self-presenting bias.
- Create an environment of trust, transparency, and open communication to ensure athletes understand the rationale for self-reporting subjective measures and the importance of doing so. The lack of education on the reasons for reporting and being unsure of the purpose and use of the data for training adaptations may impact their ability to report daily and accurate data (5).
- Give constant feedback. Without receiving feedback or seeing any improvement in training, athletes start to view the process as burdensome and with no reason to continue to do so, and therefore reduce interest, being a barrier to accurate and honest reporting.
Conclusion
- Although HRV-guided training demonstrated to be effective for training adjustments on a daily basis, the self-reported measures of stress tolerance have shown to be a promising tool. These provide more context to the HRV metrics, especially during periods of a significant increase from baseline. Thus, leading to better timing in the programming of training intensities.
- A multifaceted approach that considers both objective (HRV) and subjective (DALDA questionnaire) measures of stress tolerance is crucial for the correct interpretation of training and non-training stressors that might arise and their influence on the training process.
It is important to note that self-reported measures of stress tolerance are one more tool in the coach’s toolbox that could be used for monitoring and individualization purposes. However, it is not the only one. Therefore, it needs to be incorporated alongside with other training, and performance metrics for a better understanding of the complex interaction between training and non-training environments.
About the author
My name is Diego Hilgemberg Figueiredo. I am a sport scientist who recently completed a PhD in human performance and physical activity at the State University of Maringá, Brazil with the thesis title: “Comparison between heart rate variability and DALDA questionnaire as an instrument of endurance training prescription and their effect on 5km running performance”. I also worked as a research visitor at the High Performance and Exercise Physiology Clinic at the University of South Australia, Adelaide, under the supervision of Dr Clint Bellenger. I love science and especially its application in helping athletes to achieve better performance. You can follow me on Twitter @diegohilgemberg.
References
- Baldwin W, Williams JM. Athletic injury, psychological factors and perceptual changes during stress. J Sports Sci 17(9): 735-741, 1999.
- Buccheit, Monitoring training status with HR measures: do all roads lead to Rome? Front Physiol 5:73, 2014.
- Buchheit M, Laursen PB. High-intensity interval training, solutions to the programming puzzle: Part I: cardiopulmonary emphasis. Sports Med 43(5):313-338, 2013.
- Capostagno B, Lambert MI, Lammberts LP. Analysis of a submaximal cycle test to monitor adaptations to training: implications for optimizing training prescription. J Strength Cond Res 35(4): 924-930, 2021
- Coventry M, Timler A, Mosler AB, Russell K, Travers M, Oam LM, Murphy MC. “I lie a little bit.” A qualitative study exploring the perspectives of elite Australian athletes on self-reported data. Phys Ther Sport 60:91:97, 2023.
- Coyne JOC, Coutts AJ, Newton RU, Haff GG. The current state of subjective training load monitoring: follow-up and future directions. Sports Med Open 8(1), 2022.
- Decroix L, Lamberts RP, Meeusen R. Can the Lamberts submaximal cycle test reflect overreaching in professional cyclists? Int J Sports Physiol Perform 13(1):23-28, 2018.
- Figueiredo DH, Figueiredo DH, Bellenger C, Machado FA. Individually guided training prescription by heart rate variability and self-reported measure of stress tolerance in recreational runners: effects on endurance performance. J Sports Sci 40(24):2732-2740, 2022.
- Halson S. Monitoring training load to understand fatigue in athletes. Sports Med 44:139-147,2014
- Javaloyes A, Sarabia JM, Lamberts RP, Moya-Ramon M. Training prescription guided by heart rate variability in cycling. Int J Sports Physiol Perform, 29: 1-28, 2018
- Javaloyes A, Sarabia JM, Lamberts RP, Plews DJ, Moya-Ramon M. Training prescription guided by heart rate variability vs. block periodization in well-trained cyclists. J Strength Cond Res 34 (6): 1511-1518, 2020.
- Jeffries AC, Wallace L, Coutts AJ, McLaren SJ, McCall A, Impellizzeri FM. Athlete-reported outcome measures for monitoring training responses: A systematic review of risk of bias and measurement property quality according to the COSMIN guidelines. Int J Sports Physiol Perform 15 (9):1203-1215, 2020
- Meeusen R, Duclos M, Foster C, et al. Prevention, diagnosis, and treatment of the overtraining syndrome: joint consensus statement of the European College of Sport Science and the American College of Sports Medicine 45(1):186-205, 2013
- Nuutila OP, Nummela A, Korhonen E, Hakkinen K, Kyrolainen H. Individualized endurance training based on recovery and training status in recreational runners. Med Sci Sports Exerc 54(10):1690-1701, 2022.
- Plews DJ, Laursen PB, Stanley J, Kilding A, Buchheit M. Training Adaptation and Heart Rate variability in Elite Endurance Athletes: Opening the Doors to Effective Monitoring. Sports Med 43(9): 773-781, 2013.
- Rushall B. A toll for measuring stress tolerance in elite athletes. J App Sports Psychol 2(1):51-66, 1990.
- Saw AE, Main LC, Gastin PB. Monitoring athletes through self-report: Factors influencing implementation. Journal of Sports Science and Medicine 14 137-146, 2015
- Saw AE, Main LC, Gastin PB. Monitoring the athlete training response: subjective self-reported measures trump commonly used objective measures: a systematic review. Br J Sports Med 50(5):281-91,2016
- Shushan T, McLaren SJ, Buchheit M, Scott TJ, Barrett S, Lovell R. Submaximal fitness tests in team sports: A theoretical framework for evaluating physiological state. Sports Med 52(11):2605-2626, 2022.